Sabermetrics Primer
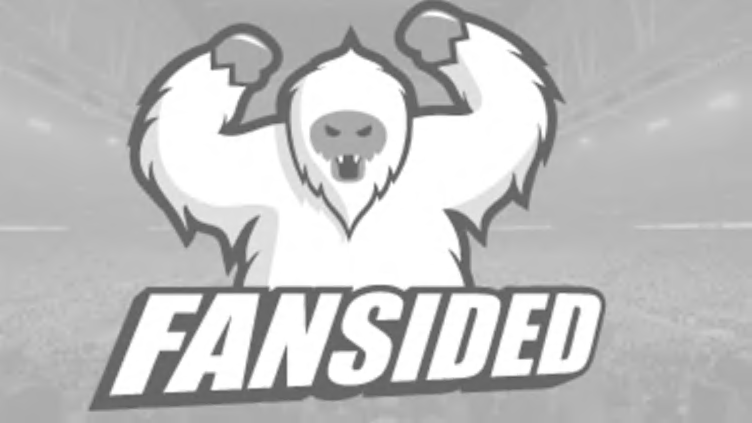
I must admit it, I’m one of “them” – a believer in sabermetrics. All that math mumbo-jumbo, as people are wont to call it, just happens to make a lot of sense to me.
It wasn’t always that way. Growing up, I was always taught that you can see everything you need to see on the baseball field with your eyes, and that numbers were just that: numbers. I learned that a .300 batting average meant that ‘player X’ was a good hitter. Anyone who wins 12-16 games with an ERA under 4.00 is a good pitcher.
More from That Balls Outta Here
- 11 Free-agent deals the Philadelphia Phillies wish fell through
- Phillies-Mets owners’ rivalry grows after shocking Carlos Correa deal
- Could Rich Hill become ‘Jamie Moyer 2.0’ in Phillies rotation?
- Does Bailey Falter have a future in Phillies’ rotation?
- Prospect Andrew Baker could help Phillies bullpen in 2023
I was young and impressionable then. I believed it all. I used this knowledge to collect baseball cards of the players who had the good “counting stats” on the back, such as homeruns and stolen bases, and began to sit back and think about the rewards these cards would give me once I sold them for a king’s ransom one day. After all, who wouldn’t want a Gregg Jefferies rookie card? Dude hit .300 a couple of times. He MUST be a star!
Once I entered college, I began reading more and more about baseball. As were many others, I was introduced to sabermetrics by the book “Moneyball”. I knew then, as I know now, that the book was more about finding inefficiencies in the free agent market.
But the way that Billy Beane went about it fascinated me. I wanted to know more. Then, like a sadist who pops balloons of small children at a circus just to see them cry, another book that I read completely blew my age-old counting stats beliefs out of the water.
It’s called “Baseball Between the Numbers“, written by the team at Baseball Prospectus. Reading this book opened my eyes further, showing me that there is a great need for statistical analysis. It further educated me that many of my “traditional” ideas about baseball were all wrong.
I devoured the book, and became a subscriber to the website. I was hooked. Since then, I have always viewed players through that statistical analysis lens, to better help understand why a player does what he does.
Now, I am by no means a mathematical person, but I do have a basic sense of how math works, and so that knowledge helps me to apply the math in sabermetrics a little more easily.
Few need advanced stats to see that Aumont is no big leaguer
(Photo Credit: Bill Streicher-USA TODAY Sports)
Since then, whenever I look at or research something, I always try to apply statistical analysis. I believe this process reveals the most accurate full picture of a player.
I do also continue to include the old school way of how baseball “should” be played in my evaluations, but since I’m not trained as a scout, I can’t look at a player and discuss the mechanics of what is going on.
I also understand that I don’t need stats to tell me whether a player absolutely stinks. When I watch Phillippe Aumont giving up 6 million walks (rough estimate) per 9 innings, I don’t need a stat like BB/9 to tell me he shouldn’t be in a Philadelphia Phillies uniform.
However, when you do start to apply more advanced statistics, a clearer picture of every single player can begin to come into focus. Case in point: Ben Revere.
Looking at Revere’s “old school” numbers from 2014, a grizzled Phillies fan (I won’t call them old or “old school”) would think that he had a nice year. After all, the centerfielder hit .306 with 49 stolen bases on the offensive side of the game.
Also, on the defensive side of things, even though his arm may be weak, his speed made him an asset in the field. All well and good. However, digging a little deeper, Revere’s season was actually nowhere near the star quality that those surface numbers might imply.
His OPS+ (On-Base plus Slugging) was 93, meaning that with a ‘100’ score revealing an average big leaguer, Revere was actually a below average regular. He had a .304 wOBA (Weighted On-Base Average) at the plate, which is also well below average, and accounted for -18 DRS (Defensive Runs Saved) in the field. Ben Revere is still a useful player, especially to a team like the Phillies, but he’s not even an average threat in the batting order.
That being said, I wanted to relay some of my favorite statistics. These are the ones I usually use the most as I think they are the best available, and you will frequently see me refer to them in the articles that I produce here at TBOH. For those who really want to research more, I provided a link for more information as to their explanations.
The great Trout-Cabrera debates in 2012 & 2013 helped grow appreciation of advanced stats.
rWAR – Most people, at this point, are familiar with at least the concept of WAR. The great Mike Trout vs Miguel Cabrera debates over who should have been the MVP in 2012 and 2013 brought the stat to the forefront of baseball terminology.
For those who aren’t familiar, WAR (Wins Above Replacement) in its most basic form is the number of Wins that a specific player would contribute over what, if that player were out of the lineup, a replacement player would produce instead.
It takes into account all facets of a player’s game, and turns those into a nice, tidy number. While many would consider WAR to be a flawed stat (and I think it is), it’s the best thing we have now to compare not only players in today’s game, but players across different eras of history (WAR takes into account playing conditions, scoring environment, ballparks, etc.).
There are two versions widely used: rWAR (Baseball Reference’s version) and fWAR (Fangraphs‘ version). Each resource calculates the number a little differently, but not so much as to have a wide difference of opinion on any given player. I prefer rWAR simply because I use Baseball Reference more frequently. For a more detailed explanation, click here.
wOBA – Here is a link to a very detailed explanation of this stat, since it’s very hard to sum up quickly. Take your time and read it, I’ll wait.
….done?…..
Welcome back. I like the wOBA stat because it paints a clearer picture of a batter’s actual offensive value. This and wRC+ should give a fan a better picture of who is the best hitter on a particular team, within a division, or across an entire league.
FIP – FIP (Fielding Independent Pitching) strips away everything a pitcher is not personally responsible for, such as errors and other miscues by defenders, and presents his true pitching ability to look like his ERA. In other words, the number reads like ERA, where under 3.50 is a good pitcher, under 3.00 is a great pitcher, and over 4.00 is someone who is average or worse.
Voros McCracken’s work broke ground in advanced stats analysis
ERA is so defense-dependent that it makes it difficult for one to see if a pitcher is truly any good. Included in the link inserted above, there is research done by the great Voros McCracken that suggested a pitcher isn’t responsible for much once the bat meets the ball since he can only control certain things (the whole piece is worth reading, and is considered one of the turning point pieces of research in baseball analysis). If one uses FIP, it’ll show a clearer picture of how good a pitcher actually is.
DRS – This stands for “Defensive Runs Saved” in the sabermetrics community. Defensive metrics are more recent in their studies, as they require more daily viewing in order to see and calculate correctly. However, with the help of modern technology, including the fact that every game is televised and can therefore be recorded and studied, analyzing a quantifying defensive contributions is becoming very accurate.
This article has been intended as a very basic introduction to those who might not be familiar with the newer stats available, but who may have heard of them, and who may be interested in learning more. It is well worth your time to investigate further and try to get a grasp on these stats.
Front offices in the game are already using these numbers to make major decisions. Even the Phillies, who were in the statistical Stone Age prior to 2014, have begun to come around to using these stats to make informed decisions.
While I can’t convince you to adopt sabermetrics entirely, I’d encourage that if you love the game of baseball, to expand your mind and try to use them. I can almost guarantee that it will make your viewing of our great game a little more enjoyable.